Learning with AI: Learning Strategies
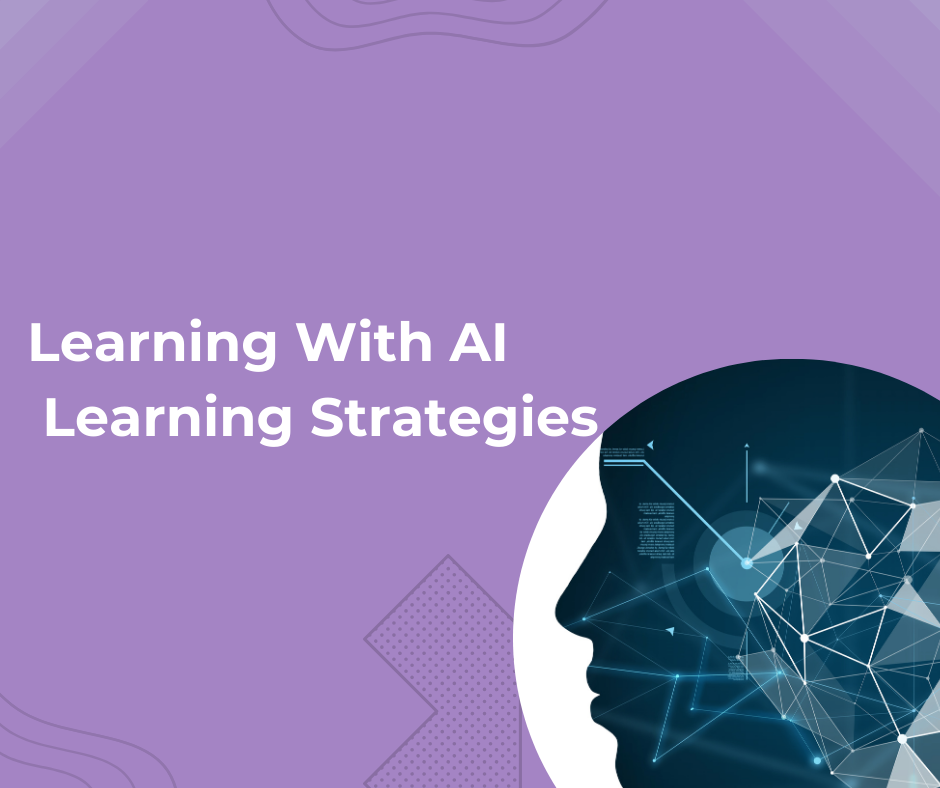
"In the rush toward focus on the word 'experience' the word 'learning' can get lost." - Donald Clark.
This quote has been with me ever since I read Learning Experience Design: How to Create Effective Learning that Works (1) a few years ago. It highlights a critical tension in modern educational design: the risk of prioritising novel experiences over actual learning outcomes. In my previous roles, I've witnessed this trend firsthand and have had to fight hard to keep learning a priority—sometimes winning these battles, sometimes watching helplessly as flashy features and AI-first approach trump fundamental learning principles.
In this article, the term AI refers to AI tools students mostly use (2): ChatGPT, Grammarly, Microsoft Copilot, Google Gemini, and Perplexity
Artificial intelligence enters this equation balancing learning and experience as both an enabler and a potential distraction. It can personalise learning paths, provide immediate feedback, analyse performance patterns, and deliver content in adaptive ways that traditional approaches cannot match. However, AI implementation in education also risks becoming just another "experience" that overshadows learning if not thoughtfully integrated.
In our previous articles in this series, we've been exploring the learning process and AI's impact on it.
In the first article, Learning with AI - Introduction, we introduced the three phases of learning: encoding, storage, and retrieval, described the forgetting curve, and discussed how overuse of AI tools can cause cognitive atrophy.
The second article, Learning with AI - Encoding, focused on elaboration techniques that help us understand and connect with new information, noting how over-reliance on AI tools can lead students to skip crucial elaboration steps that build strong neural connections.
The third article, Learning with AI - Storage, explored how information moves from working memory to long-term memory and why the timing of study sessions matters more than their duration.
Our fourth article, Learning with AI - Retrieval, examined the phase when we need to access and use stored information, highlighting how retrieval strengthens memory pathways and how AI tools can either support or short-circuit this essential process.
This final article focuses on the strategies employed by effective learners and examines how AI can enhance these strategies while avoiding the pitfall Clark warns about: losing sight of learning itself.
As we wrap up our introduction, let's have a look at how AI is marketed to students versus how learning actually works. AI tools are often positioned as features that make learning easier—they can instantly summarise complex texts, automatically generate flashcards, solve problems step-by-step, and provide immediate answers to virtually any question. Technology companies, many schools and universities highlight how these tools can save time and reduce cognitive load. Students are promised they can "work smarter, not harder" by delegating these mental tasks to AI assistants.
This message is certainly appealing. Who wouldn't want to bypass the struggle of learning? Why wrestle with difficult concepts when an AI can explain them clearly? Why create your own study materials when an AI can generate them for you? Why think through a problem when an AI can solve it instantly?
But this is where we encounter a disconnect between how AI tools are promoted and what cognitive science tells us about effective learning. Research by Biwer and colleagues (3), along with decades of studies on learning and memory, reveals a counterintuitive truth: successful learning should be difficult.
This brings us to our first section, where we'll explore the concept of "desirable difficulties" and why the very aspects of learning that feel challenging are often the most crucial for building durable knowledge.
"Desirable Difficulties" in Learning
Effective learning strategies rely heavily on "desirable difficulties," a concept that many students find counterintuitive. As Biwer and colleagues explain in their research, desirable difficulties refer to learning conditions that initially make the learning process more challenging and effortful but lead to stronger long-term retention and transfer.
Simply said, learning works in a counterintuitive way: strategies that feel easy lead to forgetting, while strategies that feel difficult lead to remembering.
The study mentioned above found that most students tend to rely on passive, ineffective strategies such as rereading and highlighting. These approaches create a misleading sense of mastery because they make the learning process feel smooth and straightforward. As a result, students often overestimate how much they've actually learned and are surprised when this information isn't readily accessible during exams.
Learning Strategies that Work
Writing summaries, rereading, self-explanation, highlighting, practice testing, distributed practice... Which of these strategies do learners mostly use? And which of these really work?
Dunlosky and colleagues (4) provided thorough reviews of 10 commonly used learning strategies and identified how effective they are for long-term learning.
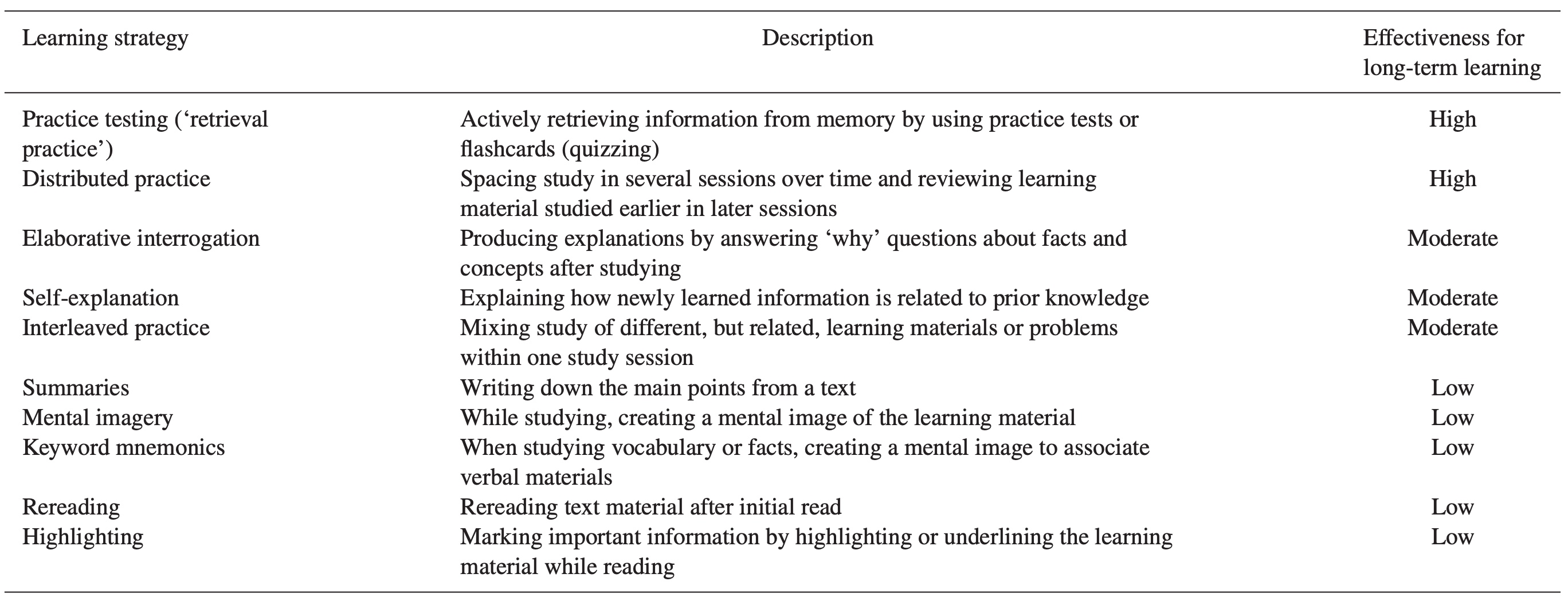
In the following text, we'll examine learning strategies that research has proven to be highly or moderately effective. For each strategy, you can find practical suggestions on how to leverage AI tools to support—rather than shortcut—the cognitive work these strategies promote.
1. Retrieval practice (practice testing): Instead of simply rereading information, actively retrieving it from memory through self-quizzing creates a productive struggle that strengthens memory pathways. The researchers found this to be among the most effective strategies for long-term retention.
How to use AI?
📝 Quiz generation prompt: "Create 10 quiz questions about [topic] that test my understanding at different levels. Don't provide any tips or answers immediately."
✍ Recall prompt: "This is my explanation of the key concepts of [topic] from memory. Identify any gaps or misconceptions in my understanding."
2. Distributed practice: Spacing out learning over time rather than cramming creates beneficial forgetting between sessions, requiring more effort to recall information but ultimately strengthening retention.
How to use AI?
📝 Spaced review prompt: "I need to learn [topic] over the next two weeks. Create a study schedule that spaces out my practice sessions (starting close together, then gradually farther apart). For each session, suggest specific ways to test myself on what I've learned."
✍ Revision reminder prompt: "Generate 3 surprise quiz questions about [topic I studied 2 weeks ago] to test how much I remember."
3. Interleaved practice: Mixing different topics or problem types within a single study session feels more difficult than blocked practice (focusing on one topic at a time) but leads to better discrimination between concepts and improved transfer.
How to use AI?
📝 Topic mixing prompt: "Create a study session that interleaves practice questions from these three related topics: [topic 1], [topic 2], and [topic 3]. Don't group the questions by topic."
✍ Switching context prompt: "Create a sequence of questions that forces me to switch between [multiple concepts] without signalling which concept each question relates to."
4. Self-explanation: Instead of just reading material passively, explaining concepts in your own words by connecting them to what you already know helps build stronger understanding. When you actively explain "why" something works or makes sense, you create more meaningful connections in your brain.
How to use AI?
📝 Process explanation prompt: "Present a problem about [topic], and ask me to explain my thinking as I work through it. Then tell me what I missed or misunderstood."
✍ Concept breakdown prompt: "After I explain [concept] in my own words, point out any parts that are unclear or incomplete."
5. Elaborative interrogation: Asking yourself "why" and "how" questions while studying helps you think more deeply about the material. Rather than accepting facts at face value, questioning helps you understand underlying reasons and creates stronger memory connections.
How to use AI?
📝 Why-questioning prompt: "For these key points about [topic], ask me why each one is true or important, and have me explain the reasoning."
✍ Connection prompt: "Ask me to explain how [new concept] relates to [something I already know] and why this connection matters."
After examining these effective learning strategies - practice testing, distributed practice, and elaborative interrogation - it's important to note that these are all cognitive strategies, focusing directly on how information is processed and remembered. Yet there's another category of strategies that guides our decisions about which cognitive approaches to use and when: metacognitive strategies, which direct us in thinking about our own thinking.
Metacognitive Strategies in Learning
While cognitive strategies focus on the direct processing of information, metacognitive strategies govern how we select, monitor, and evaluate those cognitive processes.
As Stanton, Sebesta, and Dunlosky (5) define it, metacognition is "awareness and control of thinking for learning." This concept encompasses two critical components:
metacognitive knowledge - understanding your own thinking and approaches to learning and
metacognitive regulation - actively controlling your thinking through planning, monitoring, and evaluating
Students with well-developed metacognition can identify concepts they don't understand, select appropriate strategies for learning those concepts, implement the strategies effectively, and adjust their approach based on outcomes. Furthermore, they can better decide when and how to use AI tools productively, recognising both how these tools can enhance their learning process and when over-reliance might short-circuit the necessary cognitive challenges that build durable knowledge.
Why can these learners do this? Because they actively think about their own thinking and learning processes, rather than passively consuming information—they employ their metacognition to maintain control over their learning journey, including their use of technological tools.
The relationship between cognitive and metacognitive strategies is symbiotic. Cognitive strategies like retrieval practice, spacing, and interleaving provide the tools for effective learning, while metacognitive strategies help learners decide when and how to use these tools most effectively. For example, a student might employ self-testing (a cognitive strategy) after recognising (metacognition) that passive reading isn't yielding adequate understanding. Similarly, they might choose distributed practice over cramming after evaluating the long-term effectiveness of their previous study methods.
When learners become aware of the learning strategies they're using, monitor how effectively these strategies are working, and make adjustments based on that assessment, they develop the autonomy and self-regulation needed for lifelong learning. This integration of how to study (cognitive) with understanding why to study in a certain way (metacognitive) creates a powerful framework for more efficient and effective learning in any context.
However, the learning strategies we've discussed probably sound unfamiliar to most learners. Let's face it - terms like "distributed practice" and "metacognitive regulation" don't typically come up in everyday conversation. This creates a practical challenge. It's really up to schools and universities, instructional designers, and learning experience teams to build these principles into courses and materials without expecting students to figure it all out on their own. When we integrate AI tools into education, we need to design experiences that support real learning rather than just offering easy shortcuts, even if students don't recognise the learning science happening behind the scenes.
AI in Learning
Compare these two scenarios and think about the quote from the beginning "In the rush toward focus on the word 'experience' the word 'learning' can get lost."
Example 1
A learning platform introduces an "AI Study Buddy" feature with marketing that promises: "No time to read? Let our AI do the heavy lifting! With one click, generate perfect summaries, solved problems, and ready-to-submit answers. Why struggle when AI can think for you?"
The interface prominently displays buttons labeled "Instant Summary," "Solve This Problem," and "Generate Essay" at every challenging point in the learning journey. When students encounter difficult material, the AI immediately suggests, "This looks tough! Would you like me to explain it for you?" before the student has attempted to understand it themselves. The design celebrates efficiency and ease rather than learning effectiveness, treating struggle as something to be avoided rather than embraced.
Example 2
A learning experience introduces "AI Learning Coach" with different messaging: "Develop stronger understanding through guided reflection. Your AI coach helps you think more deeply about concepts and monitor your learning progress."
In this implementation, the AI first asks students to articulate their current understanding before offering any assistance. When a student encounters a challenging concept, instead of immediately providing an explanation, the AI asks: "What parts of this concept are clear to you, and what aspects seem confusing?" After the student attempts a problem, the AI prompts reflection: "What strategy did you use to approach this problem? How confident are you in your solution?"
The interface is designed with intentional friction—AI help is available but requires the student to engage in planning, monitoring, and evaluation. For example, before generating a summary, the AI asks the student to identify key points they've already understood and areas they found confusing, then uses this input to provide targeted guidance rather than replacing the student's cognitive work.
Which example is about the experience and which one focuses on learning?
Conclusion
As we close this fifth and final article in our Learning with AI series, we circle back to Donald Clark's insight about experience potentially overshadowing learning. The research is straightforward: good learning often feels challenging. The most effective approaches—testing yourself, spacing out practice, mixing up topics, and thinking about your own learning—create beneficial struggles that many features, including AI, might be designed to eliminate.
AI tools can either become shortcuts that do the thinking for students, or thoughtful partners that strengthen how students learn. The difference isn't in the technology—it's in how we use it.
This puts a special responsibility on instructional designers and learning experience teams. By building proven learning strategies into courses and guiding how AI is used, we can help students develop not just subject knowledge, but learning skills that last a lifetime. The goal isn't to make education effortless, but to make it effective—even as AI becomes more integrated into how we learn.
In this series, we've explored how AI impacts encoding, storage, retrieval, and now learning strategies. The message throughout has been consistent:
AI offers powerful support for learning when used wisely, but it's most valuable when it enhances rather than replaces the productive mental work that builds lasting knowledge.
Read more in:
1) Clark, D. (2021). Learning experience design: How to create effective learning that works. Kogan Page.
2) https://universitybusiness.com/these-5-ai-tools-are-the-most-popular-among-students/
3) Biwer, F., oude Egbrink, M., Aalten, P., B.H. de Bruin, A. (2020). Fostering Effective Learning Strategies in Higher Education – A Mixed-Methods Study, Journal of Applied Research in Memory and Cognition. 9 (2), 186-203.
4) Dunlosky, J., Rawson, K.A., Marsh, E.J., Nathan, M.J., Willingham, D.T. (2013).